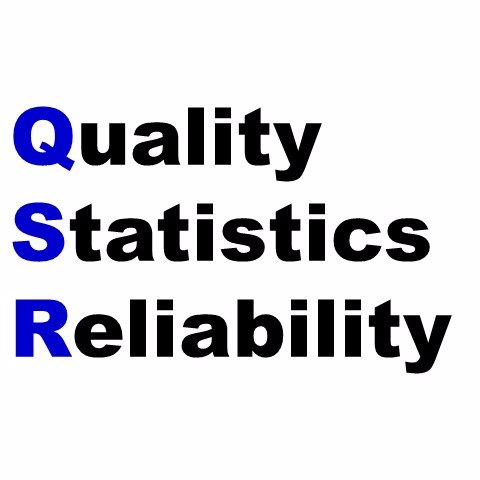
In-situ quality process monitoring in Additive Manufacturing
Call for participation
The Quality, Statistics, and Reliability (QSR) Section of the Institute for Operations Research and the Management Sciences (INFORMS) announces the Data Challenge Award to recognize excellence in data modeling techniques among the submissions to the 2021 QSR Data Challenge Competition. This award program brings prestige to the QSR Section as well as to the recipients honored.
UPDATE: In 2021, manuscripts prepared by those finalists of the QSR Data Challenge Competition who develop significant new methodologies may be considered for a fast-track review process in the INFORMS Journal on Data Science (IJDS). To prepare for this process, the participating teams should read the Editorial Statement by Professor Galit Shmueli, Editor-in-Chief of IJDS, which is available at the following two links:
https://pubsonline.informs.org/page/ijds/editorial-statement
https://pubsonline.informs.org/doi/pdf/10.1287/ijds.2020.003
Date: October 23, 2021 (to be held during the 1st QSR Workshop)
Physical Location: Anaheim, CA
Final Submission Deadline: September 12, 2021
Finalist Announcement: September 24, 2021
INFORMS Website: http://meetings2.informs.org/wordpress/anaheim2021/
INFORMS QSR Website: https://connect.informs.org/qsr/home
This year’s Data Challenge problem is sponsored by a collaboration between Politecnico di Milano (Italy) and Trumpf (Germany) fostering open data science for “In-situ quality process monitoring in Additive Manufacturing”.
Three to four finalists for the Data Challenge Award will be selected to make presentations at the First INFORMS Workshop on Quality, Statistics, and Reliability prior to the 2021 INFORMS Annual Meeting. The workshop will be held on October 23, 2021 in Anaheim, CA. The winner will be announced at the QSR Business Meeting later at the Annual Meeting. All finalists will receive an award certificate. The winner will also receive a plaque of recognition.
Eligibility Requirements:
- At least one member of a finalist team must be a QSR member.
- Team submissions (with no more than four members per team) are welcome. We especially encourage the participation of industry members on the teams.
- At least one member of each of the finalist teams must be prepared to present their work in-person during a hybrid session of the INFORMS Workshop on Quality, Statistics, and Reliability. In the unfortunate case that the workshop is to be virtually held due to COVID, we will follow INFORMS guidelines and seek alternative presentation formats (e.g., the presentations will be performed virtually and there will be no in-person component to the competition).
UPDATE: Submission instructions will be announced by August 20, 2021. Submission documents will include source codes (at this time, written only in Python) that can be run on a test set (which is not shared with the participants), and a ReadMe.docx file.
The organizers of the Data Challenge Award will host a 30-min Q&A Zoom session on August 4 th at 10 AM Eastern Time. The details of this zoom session are as follows:
Time: Aug 4, 2021 10:00 AM Eastern Time (US and Canada)
Join Zoom Meeting
https://miami.zoom.us/j/91294607417?pwd=dUZobmp1YXc3R2VuY3hraG5vUkZqdz09
Meeting ID: 912 9460 7417
Passcode: 291244
One tap mobile
+16465588656,,91294607417# US (New York)
Contacts:
Ramin Moghaddass – ramin@miami.edu
Team
Trumpf
Marc Gronle
Frederik Schaal
Politecnico di Milano, Department of Mehanical Engineering
Bianca Maria Colosimo
Marco Grasso
Emidio Granito
QSR Section
Arman Sabbaghi
Kamran Paynabar
Ran Jin
Xiaoyu Chen
Ramin Moghaddass
References
Colosimo, B. M., Huang, Q., Dasgupta, T., & Tsung, F. (2018). Opportunities and challenges of quality engineering for additive manufacturing. Journal of Quality Technology, 50(3), 233-252.
Grasso, M., & Colosimo, B. M. (2017). Process defects and in situ monitoring methods in metal powder bed fusion: a review. Measurement Science and Technology, 28(4), 044005.
Colosimo, B. M. (2018). Modeling and monitoring methods for spatial and image data. Quality Engineering, 30(1), 94-111.